How AI will Impact our Future: Lessons from the MIT AI Conference – Part I
Covering agents, big tech's positioning, the value of open source, and the future of AI models
Welcome! I'm David Martin and this is my tech newsletter. If you’ve received it, then you either subscribed or someone forwarded it to you. If you fit into the latter camp, then you can subscribe by clicking on this handy little button:
Let’s jump into it…
Build. Build. Build. The words were a frequent refrain on stage, and an apparent mantra hammered into these MIT alum. I attended the MIT AI Conference for the second time – the first time being in 2017 - and both were days well spent and packed with insight. It’s an alum conference, so the atmosphere is more cordial and relaxed than your average tech conference. It was also held on a Saturday, so the attendees were primarily there to learn. A dozen or so even made it a family affair. I am a fan – and I’ll be back next year.
Special note: I split this post into two parts to make it easier to digest. Part two is here.
In part one, we’ll cover these topics:
Agents – the next tangible step
Incumbent ‘big tech’ vs. challengers
Closed vs. open source models
The future of AI models
In part two, we’ll get into these topics and summarize the key takeaways:
The future of work
New business models
Societal impact
Summary
Agents – the next tangible step
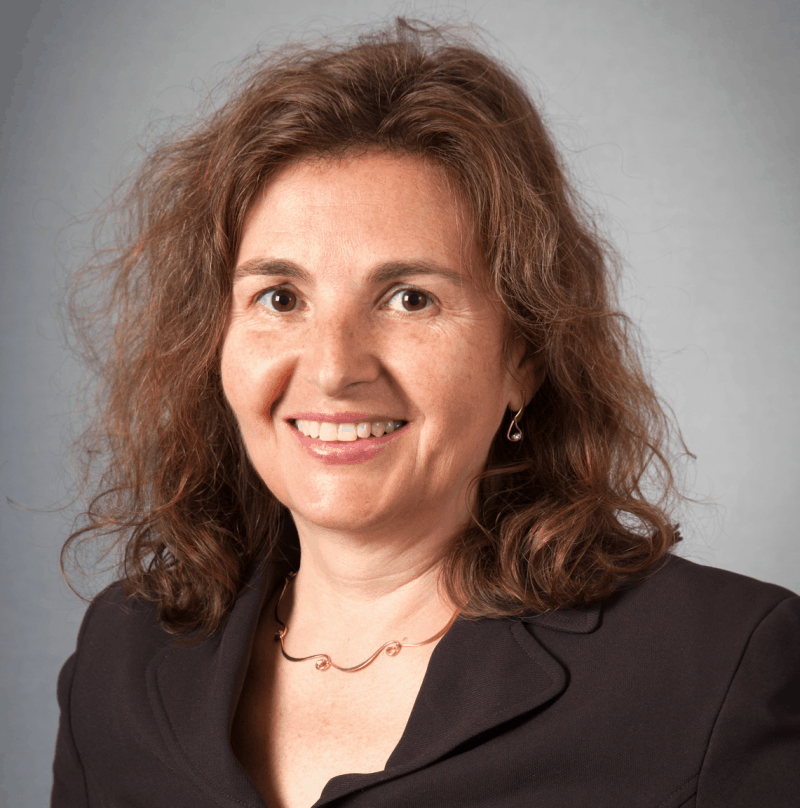
While models of the large language variety are a major tech narrative, speakers on stage also commonly use the word ’agents’ aka autonomous agents per the recent OpenAI announcements. Daniela Rus, Director of CSAIL at MIT, describes agents as assisting, augmenting, and automating. In simple terms, an agent can manage and process a task reliably. For now, it’s an aspiration.
From my personal experience, GPT-4 sometimes has trouble with more complex queries that are compute-intensive. I have noticed several startups bridge the gap with prompt ‘recipes’ and prompt management tools – some of which sit on top of GPT in the browser: AIRPRM, PromptHub, PromptFolder, and Vidura, for example. Now, thanks to OpenAI’s announcement about their app store, these startups will have to ‘pivot.’ Regardless of how they are achieved, agents will help to realize Daniela’s vision of automating tasks.
Shishir Mehrotra, CEO/Founder of CODA, covered three requirements for agents in the enterprise: 1) they need to have knowledge of the internet and your company documents; 2) they must “live where you live,” i.e., be omnipresent with you while you are working; and 3) they must manage and complete tasks which you verify before they take action (human in the loop).
Incumbent ‘big tech’ vs. challengers
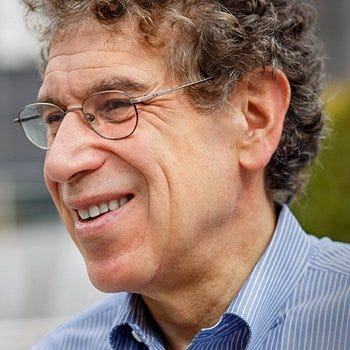
Who stands to win with AI adoption? Mark Gorenberg, Managing Director at Zetta Ventures and Chair of MIT Board of Trustees, stated that the key to this question is whether or not the user workflow changes. Incumbent tech companies are investing heavily in generative AI, but, all things being equal, if the workflow changes, then there’s an opening for startups to become the new default. Notably, the workflow has already changed: the previous generation of smart assistants (Alexa, Google Home, Siri) respond when called upon, while new versions can be ever-present (GitHub Co-Pilot video) and offer interactive conversations (ChatGPT Plus video).
Two examples point to even further changes: yesterday, humane.ai launched their AI Pin or ‘ambient computing’ device. The startup has been in the news with $100M in funding and a new device category that feels “familiar, natural, and human.” Imran Chaudri, ex-Apple, and his partner Bethany Bongiorno want AI to make our devices disappear and showed off an early demo in an April TED Talk. Similarly, Jony Ive, Apple’s former Chief Design Officer, and his firm LoveFrom are in talks with OpenAI to build an AI-first phone. In both cases, the workflow could fundamentally change, allowing for a tectonic shift in the tech landscape.
Closed vs. open-source models
While not a new question, proponents of open source argue that making these models (Meta’s Llama, Anthropic’s Claude, Hugging Face’s 380k models) publicly available enables crowdsourcing of researchers to vet models and adjust for biases – contending they are more transparent. Opponents counter that companies with proprietary models (OpenAI, Microsoft, and Alphabet) will react to public pressure on specific issues to maintain their brands and stock prices. Also, because of their profit motive, they are incentivized to invest in improving their models, reducing biases, and delivering a better experience. Common wisdom also says that proprietary models are less likely to be used for malicious purposes such as hacking or misinformation: the idea that Vladimir Putin could use open-source models to sway future elections came up more than once at the conference.
Greg Brockman’s ‘ratcheting’ answer to this question is nuanced and, definitively, a ‘yes’ to both open-source and proprietary models. If I read between the lines correctly, his vision for the future implies that the proprietary models may play a role in counteracting some of the adverse effects of having powerful open-source models out in the wild.
“I think the emerging story we’re seeing is actually quite good, to the point of frontier models that are going to be, you know, some hundred years ahead, and it’s going to be open-source models that are going to be quite capable as well, we’re going to be ratcheting, we’re going to have time to sort of adjust and see what the impacts are and how to mitigate them.”
- Greg Brockman, President, Chairman, & Co-Founder, OpenAI
The future of AI models
Today, ChatGPT can now see, hear, and speak. LLMs and computer vision models are buzzing in the tech scene, but how can they evolve, and what about other non-language and computer vision models?
Entrepreneurs have plenty of gaps and opportunities to fill.
Mark Gorenberg, Managing Partner at Zetta Ventures and Chair of MIT Board of Trustees, discussed these gaps and how models lack reasoning, frequently hallucinate, sometimes stumble over math, and are compute-hungry. Today, they also feature significant latency that makes them ill-suited to real-time applications.
Elad Gil, investor and former tech executive, mentioned his recent conversation with Ilya Sutskever, Chief Scientist at OpenAI. Ilya believes the transformer model may apply to other forms of human cognition beyond natural language. A team from Alphabet and the University of Toronto initially devised the transformer in 2017. This deep learning architecture enables generative AI models to understand questions by understanding word order (positional encoding) and how a word fits in the context of the words surrounding it (self-attention) – among other features.
Kanjun Qiu, CEO & Co-Founder, talked about Imbue’s “CARBS,” aka Cost-Aware pareto-Region Bayesian Search that optimizes model selection based on the task and carbon footprint. Named after her co-founder, Josh Albrecht’s love for the food, CARBS finds the highest performing model for a specified amount of compute. If adopted, this approach could imply a potential “meta” architecture to agents whereby they select a model based on specific criteria such as whether or not you’re a paid user, your environmental preferences, the difficulty of the question, and the compute available.
I especially thank Ken Yeung and Katy Hunt for their feedback and advice on these posts and the newsletter.
Want to learn more? Check out part two.